Real data, real problems - students apply data science in practice
Quality control in steelworks, stress in studies and diversity in marketing - analysing large amounts of data brings added value in very different fields. Students were able to see this for themselves in the "Data Science & Decision Support" project seminar run by Prof Dr Charlotte Köhler in the winter semester 2024/25. They worked on real data and problems in three groups and learnt a lot about time management and group work along the way.
"Working with real-world data is far more complex and nuanced than working with simulated or curated datasets, which are often clearer and more structured," says Marydaphine Ochoi, summarising one of her most important experiences from the seminar. She is studying International Business Administration (IBA) in her fourth semester and took part in Charlotte Köhler's seminar, where she tackled real-life challenges at the steelworks in Eisenhüttenstadt. Her group, which also includes Chefor Ndah Akofor, Felipe Oliveira and Gisela Winda Sutanto, received data from Arcelor Mittal. There, the problem repeatedly arises that machine monitoring of weld seams produces different results than inspection by a human employee. But when do these discrepancies occur? What leads to the different assessment? To get closer to the answer to this question, the students analysed the data and found a pattern, as Marydaphine Ochoi explains: "For certain coil thicknesses, we consistently found only one type of discrepancy, where the machine approved the weld but the human operator did not. We think this pattern is particularly valuable, and this specific area can be investigated further and help eliminate this type of discrepancy."
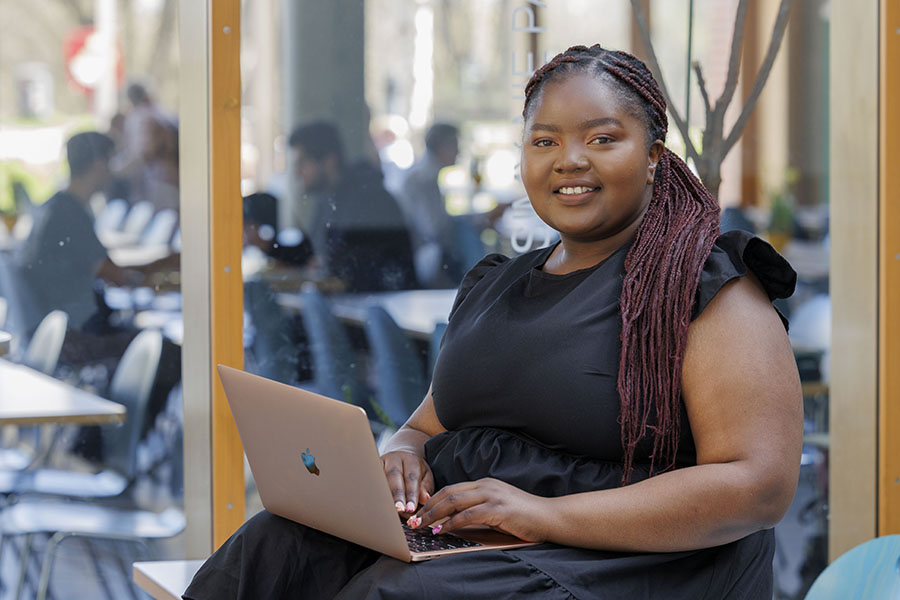
Student Marydaphine Ochoi
Heide Fest
Stress of students measured with sensors
A second working group in the seminar tackled a particularly obvious problem: They investigated the stress of Viadrina students. In addition to existing data from large-scale surveys, they also analysed the stress levels of fellow students using questionnaires and sensors for heart rate and sleep rhythm. "Our passive and active data show that exam-related stress is significant," is one of the conclusions in the group's final presentation. There is also a connection between sleep, caffeine, exercise and stress, but this needs to be analysed further. The group includes IBA students Edvin Davis Pozhaliparambil, Mahmoud Anas Khalgui, Simran Dewnani, Udval Oyunsaikhan, Anish Joseph and Ani Hovhannisyan. After the seminar, they all agreed that they had learnt a lot about effective teamwork as well as practical hard skills in data analysis.
Seminar focuses on flexibility and individual support
This observation is shared by seminar leader Prof Dr Charlotte Köhler, holder of the Professorship of Business Administration, in particular Business Analytics. "Group work is not always easy, as it requires a lot of coordination - especially if the team members have only just got to know each other in the seminar. However, all the groups managed to identify their strengths and weaknesses and utilise these in a targeted manner for the success of the project," says Charlotte Köhler. In her seminar, she deliberately favours great flexibility in working on the problems, coupled with fixed deadlines and individual support. "The students work independently on their projects, reflect on challenges and develop solutions that convince both the cooperation partners and the evaluating teachers," she says, outlining her concept. She was particularly impressed by the creativity in the results of the group projects and the way they dealt with challenges.
Seminar leader Prof Dr Charlotte Köhler
This also applies to the work of a third group, which investigated the comparison between artificial intelligence (AI) and human expertise in recognising gender and ethnicity in photos from advertisements. The starting point was a question from marketing research: the inadequate presence of certain population groups in advertising and their often stereotypical depiction. Until now, the analysis of such advertising images has mostly been carried out manually - a time-consuming and subjective process.
Training data from AI models critically scrutinised
Students Rose Dennis, Mukhammad Egamkulov, Litta Jose Thottam, Valeriia Knurova and Hong Minh Nguyen investigated the question of how reliably current AI models can perform this task. Unsurprisingly, the artificial intelligence recognised white, adult men particularly reliably. For people with other characteristics - such as a different gender, ethnic background or age - the recognition accuracy dropped significantly. One possible reason: unbalanced training data used to train the models. For example, one model analysed was based exclusively on photos of celebrities - a group that does not adequately reflect social diversity.
The group's conclusion: "Neither AI nor humans are better. While AI can quickly process large datasets and apply consistent rules, it struggles with subtle details and diverse or unclear data due to biases. In contrast, human experts can adapt better to context and complexity, though they may be inconsistent." Charlotte Köhler found it particularly commendable that the group critically scrutinised the training data of the AI models and was therefore able to make a well-founded assessment of the results.
The students agree that the seminar was particularly instructive for them, regardless of the subject matter. Marydaphine Ochoi says: "This project seminar reaffirmed that the knowledge we acquire in Viadrina is highly relevant and essential in real-world applications."
Frauke Adesiyan, translated by Deepl and edited
Back to the news portal
Share article: